The realm of scientific research has always thrived on human curiosity and intellect, pushing boundaries to unveil the unknown. The latest buzz in the field revolves around a groundbreaking development – the “AI scientist.”
This innovative concept promises to revolutionize how scientific discoveries are made by harnessing the power of artificial intelligence. But what exactly does this entail, and how might it transform the landscape of scientific inquiry?
Understanding the Role of an AI Scientist
An AI scientist represents a leap forward in automation within scientific research. This system aims to mimic the intricate processes of scientific discovery—from identifying research gaps and formulating questions to conducting experiments and analyzing results. Recently, Sakana AI Labs introduced their AI scientist, which they claim can autonomously perform these tasks, including drafting and publishing research papers.
The AI Scientist’s Workflow
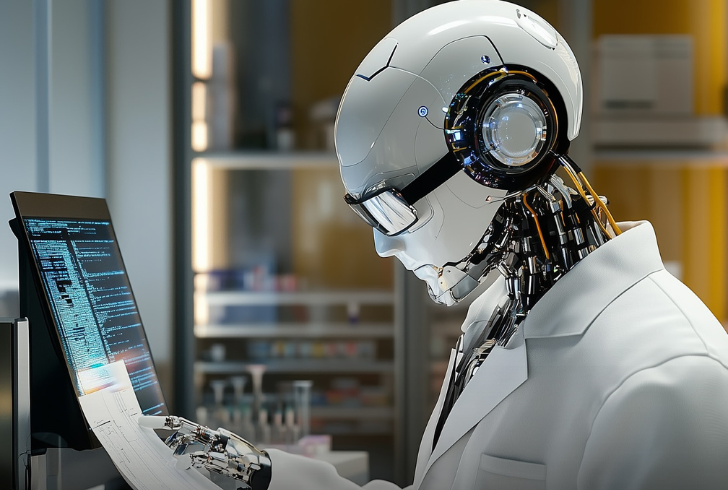
Here’s a look at how Sakana’s AI scientist operates:
- Idea Generation – Utilizing large language models (LLMs), the AI generates novel research ideas based on existing scientific literature.
- Algorithm Development – The system codes new algorithms tailored to test these ideas.
- Experimentation – Results are plotted and analyzed using AI-driven tools.
- Documentation – A comprehensive research paper is drafted, summarizing findings and including relevant references.
This entire process is reportedly achieved at a minimal cost of $15 per paper, a stark contrast to the significant expense associated with traditional scientific research.
Can AI Truly Innovate?
While the AI scientist is designed to produce research that mirrors human scientific inquiry, its ability to generate truly innovative ideas remains under scrutiny. The system uses extensive data from scientific repositories, allowing it to produce content that resembles high-quality research papers. However, novelty—an essential component of impactful science—is challenging to automate.
Evaluating Novelty and Quality
To address the issue of novelty, Sakana’s AI scientist implements a dual approach:
- Similarity Scoring – New research ideas are evaluated for their uniqueness by comparing them to existing studies.
- Peer Review – Another AI system assesses the quality and originality of the generated papers.
Despite these measures, feedback on the AI’s outputs has been mixed. Critics argue that the generated papers often lack depth and innovation, describing them as “endless scientific slop.” Furthermore, initial self-assessments of these papers have highlighted their shortcomings, though improvements are anticipated as the technology matures.
AI’s Role in Supporting, Not Replacing, Scientists
Rather than replacing human scientists, AI tools aim to support and enhance their work. For example, AI-powered search and synthesis tools like Semantic Scholar, Elicit, and Research Rabbit help scientists locate and interpret relevant research efficiently. Additionally, text mining tools and machine learning algorithms assist in summarizing and comparing research findings, streamlining literature reviews.
Enhancing Scientific Efficiency
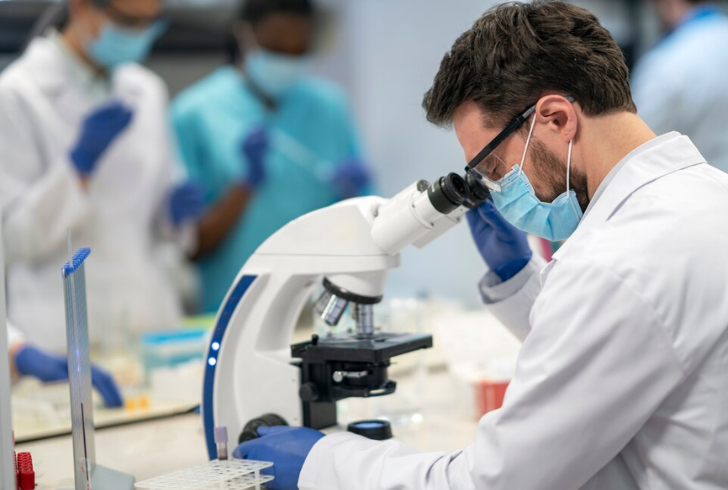
The goal of these AI tools is to augment human capabilities, not replace them. They offer valuable support in managing the vast amount of published research and identifying key information swiftly. However, the role of the human scientist remains crucial in interpreting results, ensuring research quality, and driving innovative thinking.
Potential Challenges and Ethical Considerations
The prospect of AI-generated research raises several concerns. One major issue is the potential for a flood of low-quality papers, which could overwhelm the peer review process and diminish the overall trust in scientific literature.
Additionally, there is a risk that future AI systems might become less effective if they are trained predominantly on AI-generated content, leading to a cycle of diminishing innovation.
The Future of AI in Science
While the vision of a fully AI-driven scientific ecosystem presents intriguing possibilities, it also brings significant challenges. Ensuring the integrity of scientific research and maintaining trust in the process will be critical as AI scientists become more integrated into the field. Balancing the benefits of automation with the need for human oversight will be essential to harnessing the full potential of AI in science.